In the dynamic realm of artificial intelligence (AI), NVIDIA and AMD take the lead, challenging the limits of computational capability. Both corporations have introduced formidable AI chips, yet the comparison between H100 and MI250X prompts the question of superiority. This article will analyze performance metrics, statistics, and features of both chips to ascertain the ultimate champion in the realm of AI processing.
H100 vs. MI250X: In-Depth Side-by-Side Compariso
Category | NVIDIA H100 GPU | AMD Instinct MI250X GPU |
---|---|---|
FP32/FP64 (double) Performance | 51.22/25.61 teraFLOPS | 47.87/47.87 teraFLOPS |
Power Efficiency | TDP of 350W | TDP of 500W |
Memory | Offers 80GB at 2039GB/s | Offers 128GB at 3277GB/s |
Compatibility | Capable of seamlessly integrating with a diverse array of AI frameworks such as TensorFlow, PyTorch, and Caffe. | Optimal performance is achieved when utilized within the AMD product ecosystem. |
Price Point | ~$30,000 | ~$10,000 |
Recommendation | Remarkably efficient with robust compatibility, though at a considerably high cost. | Delivers superior performance at one-third of the price, albeit with a trade-off in efficiency. |
H100 vs. MI250X: Exploring the Distinctions
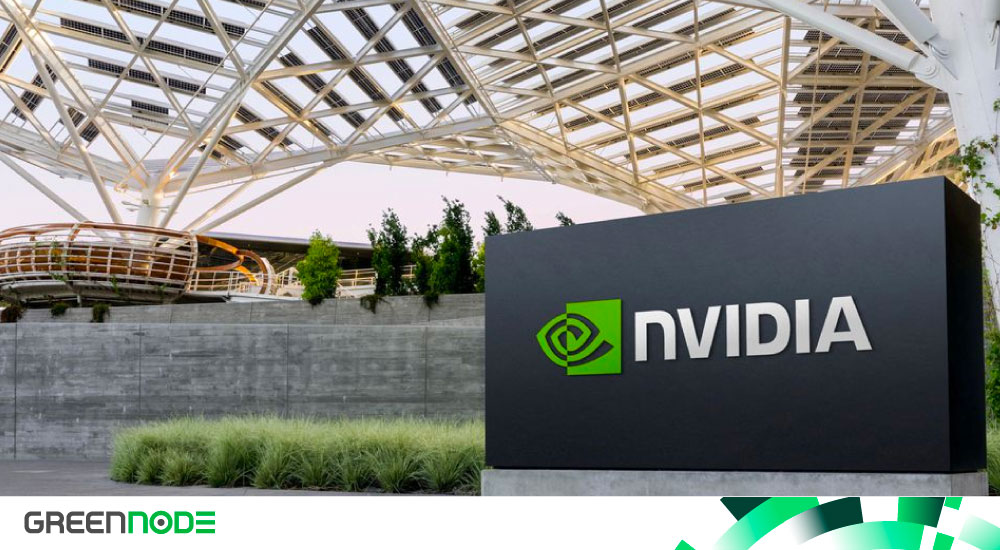
AI is no longer a technology of the future; it pervades nearly every corner of the world today. Its applications seamlessly integrate into the fabric of our daily lives, from content recommendations on streaming platforms to sophisticated medical diagnoses. At the core of this technological marvel lies the foundation of computational power, driven by specialized AI chips.
These chips were meticulously crafted by designers to effortlessly handle the computational demands of intricate machine learning algorithms - demands that standard processors would struggle to meet. Their architecture and design principles set them apart from average CPUs as they process vast amounts of data simultaneously. In essence, these chips are capable of making split-second decisions and running deep learning models with billions of parameters.
In this context, NVIDIA and AMD have introduced their flagship AI chips: the NVIDIA H100 and the AMD Instinct MI250X. Each chip represents the culmination of years of research and innovation, with both companies vying for a significant share of the AI market.
To truly grasp the power and intricacies of these chips, let's delve into six key differences: Development Histories, Performance Benchmarks, Power Efficiency, Memory & Speed, Architecture & Scalability, and Software & Compatibility.
1. Development Histories
The NVIDIA H100 GPU
Founded in 1993, NVIDIA initially gained renown for its graphics processing units (GPUs) in the gaming realm. Recognizing the potential of GPUs in AI, the company pivoted toward this emerging field. Originally designed for graphics rendering, the parallel processing capabilities of GPUs proved adept at handling the simultaneous computations required by neural networks. This realization marked NVIDIA's entry into the AI domain.
The pinnacle of this journey is embodied in the NVIDIA H100, the latest addition to the company's high-performance computing chips. With advanced architecture, including tensor cores and optimized memory management, the H100 outpaces its predecessors. It excels in both training and inference tasks in deep learning, thanks to its vast memory pool that effortlessly handles massive datasets. The H100's compatibility with leading AI frameworks ensures seamless integration for developers, while NVIDIA's extensive ecosystem, featuring platforms like CUDA and cuDNN, enhances its appeal to AI professionals and researchers.
The AMD Instinct MI250X GPU
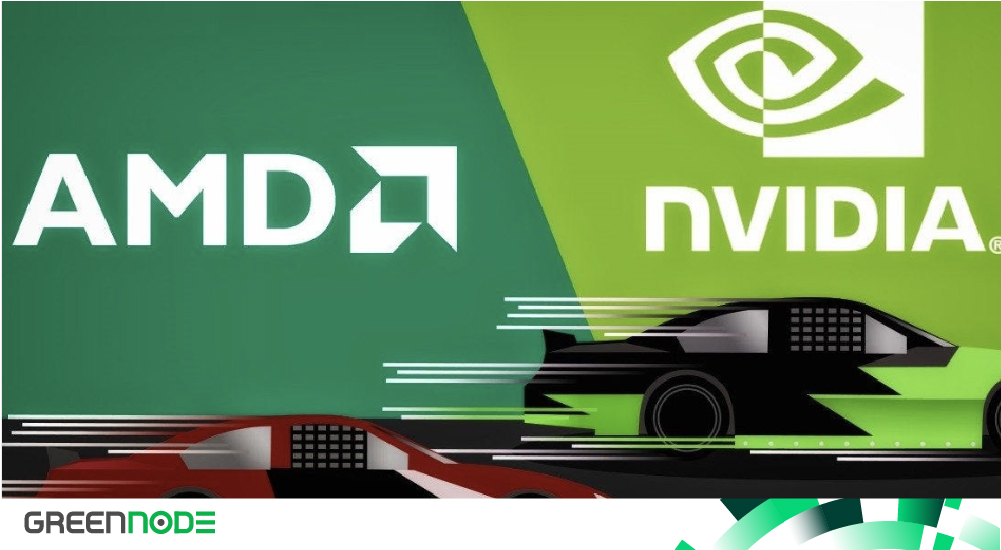
Founded in 1969, Advanced Micro Devices (AMD) emerged as NVIDIA's formidable competitor, particularly in the graphics domain. Initially focused on CPUs, AMD expanded into GPUs after acquiring ATI Technologies in 2006. Recognizing the potential of GPUs in AI, similar to NVIDIA, AMD honed its graphics cards, giving rise to the Instinct chip line tailored for machine learning and AI computations.
The AMD Instinct MI250X exemplifies AMD's dedication to advancing AI processing capabilities. Employing advanced lithography, the MI250X seamlessly combines high computational throughput with efficiency. Unlike its NVIDIA counterpart, the MI250X stands out for its smooth integration with AMD's proprietary tools, providing developers a unique blend of performance and customization. With impressive power efficiency metrics and competitive pricing, the MI250X emerges as a compelling choice for institutions and professionals seeking top-tier performance without a hefty price tag.
2. Performance Benchmarks
H100
When evaluating the performance of cutting-edge AI chips, it's essential to recognize that benchmarks provide both quantitative and qualitative insights. Raw numbers showcase computational might, while qualitative metrics reveal real-world application performance. In this context, the NVIDIA H100 stands out as a formidable powerhouse.
With its latest architectural advancements and AI-specific tensor cores, the H100 achieves impressive teraFLOPS benchmarks, indicating substantial computational capabilities. Specifically, the NVIDIA H100 GPU boasts FP32/FP64 performance benchmarks of 51.22 and 25.61 teraFLOPS, respectively. These terms denote the precision of floating-point calculations, with FP32 representing 32-bit floating-point and FP64 representing 64-bit floating-point.
The H100 excels, particularly in mixed-precision computations, crucial for tasks like training large neural networks, where speed can be prioritized without compromising accuracy. In practical scenarios, the H100 demonstrates its prowess in tasks requiring substantial parallelism.
Whether for training complex neural networks, data analytics, or simulations, the H100 consistently delivers high-performance results. Its compatibility with NVIDIA's robust ecosystem ensures top-notch software optimization, extracting maximum performance from the hardware.
MI250X
The AMD Instinct MI250X exemplifies AMD’s dedication to advancing the AI revolution. While occasionally trailing the H100 in specific metrics, this doesn't present the full picture. Across various benchmarks, the MI250X not only holds its own but frequently outperforms the H100, showcasing its versatility in diverse tasks.
A standout feature of the MI250X is its balanced performance, boasting impressive teraFLOPS benchmarks for both FP32 and FP64 at 47.87 teraFLOPS each, surpassing the H100. This ensures consistent, high-tier results for both single-precision and double-precision tasks.
Beyond numerical metrics, the chip's adaptability to real-world workloads is crucial. AMD has a history of optimizing its chips for a blend of performance and practicality, and the MI250X follows suit. Designed to handle a broad range of applications, from machine learning training to inference tasks, it stands as a testament to AMD's commitment to both performance and versatility in AI applications.
3. Power Efficiency
H100
As AI technologies advance, chip manufacturers face the challenge of striking a balance between exceptional performance and power efficiency. Power consumption considerations encompass sustainability, heat dissipation, and the long-term durability of devices. Higher power consumption often results in increased heat, necessitating more cooling, generating more noise, and potentially shortening hardware lifespans.
While NVIDIA's H100 does have its power demands, it significantly outperforms AMD's MI250X in terms of efficiency. Despite the chip's high-performance metrics, its consumption typically scales proportionately. However, NVIDIA has incorporated various power-saving techniques, ensuring greater efficiency when the chip is not under heavy load. Specifically, the NVIDIA H100 has a thermal design power (TDP) of 350W.
Manufacturers use TDP to indicate the amount of heat a component, like a GPU, must dissipate to operate within temperature limits, expressed in watts (W). In this case, the TDP of 350W for the H100 indicates that under typical workload conditions, designers expect the chip to consume and dissipate up to 350W of power.
MI250X
AMD's commitment to optimizing the power-to-performance ratio is evident in the MI250X, where every watt is meticulously engineered to extract maximum performance. However, it's worth noting that the MI250X operates at a higher wattage compared to its NVIDIA counterpart.
The elevated 500W power footprint of the MI250X results in increased heat production, demanding more robust cooling solutions. This could pose a challenge in maintaining operations within temperature limits, especially in settings such as research labs, server farms, or AI workstations.
4. Memory and Speed
H100
In the domain of AI and machine learning, memory and speed transcend mere benchmarks. These metrics determine a chip's effectiveness in handling vast datasets, executing intricate algorithms, and managing instantaneous computations - where speed plays a crucial role in project success. Initially, the NVIDIA H100 stands out for its substantial memory volume but falls short overall.
While the NVIDIA H100 boasts an impressive 80GB of onboard memory, tailored for handling expansive datasets, it is overshadowed by the MI250X in terms of raw memory size. This substantial memory proves crucial in AI tasks, especially when dealing with extensive deep learning models or neural network computations requiring simultaneous access to diverse data points.
However, storage is only one aspect; the speed at which a chip can read or write data to this memory significantly impacts performance. With a bandwidth of 2039GB/s, the H100 ensures voluminous and brisk data transfers. Yet again, the H100 lags significantly behind the MI250X in this regard.
MI250X
On the other hand, the MI250X distinguishes itself with its significantly larger memory capacity. Boasting a massive 128GB, it is tailored for a diverse array of applications, ensuring it excels in memory-intensive tasks without any compromise. This provides AMD users with nearly 50 additional GB of space to leverage.
Moreover, AMD's commitment to performance is evident in the MI250X's impressive data retrieval capabilities, featuring a remarkable bandwidth of 3277GB/s. By minimizing memory latency, the MI250X gains swift access to its extensive memory banks, outpacing competitors. This agility becomes a decisive factor in tasks requiring rapid iterations or real-time processing.
5. Architecture and Scalability
H100
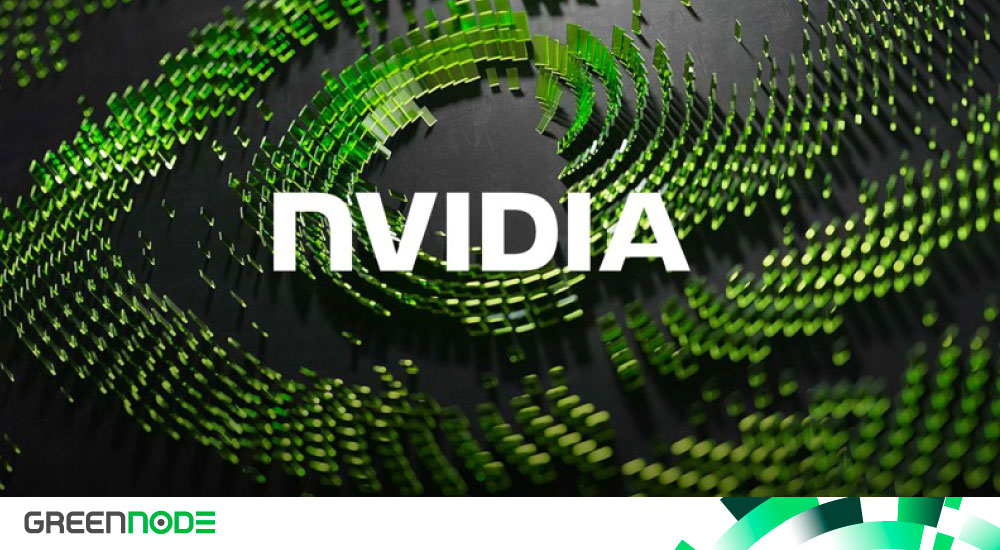
When discussing chip design, architecture serves as the blueprint for internal components and their interconnections. This aspect is crucial as it determines how efficiently a chip processes information, manages power, and communicates with other components. Additionally, a chip's architecture plays a vital role in ensuring compatibility with various software and hardware platforms.
Scalability is another pivotal attribute, especially in today's rapidly evolving tech environment. It refers to a chip's inherent ability to handle increasing workloads without a degradation in performance. In a world where clustered and parallel processing environments are common, particularly in data centers and high-performance computing, scalability is indispensable.
The H100, a flagship from NVIDIA's esteemed line, is designed around the principle of parallel processing. This method divides a task into multiple smaller sub-tasks processed simultaneously, particularly advantageous for AI computations dealing with vast amounts of data. With its forward-thinking design, the H100 ensures relevance even as AI computations become more intricate.
MI250X
The AMD MI250X exemplifies the brand's dedication to versatility and adaptability. Tailored for a spectrum of computational tasks, its architecture is optimized for the intricacies of deep learning algorithms and the demanding nature of real-time analytics.
Beyond individual performance, many computational scenarios, especially in data centers, involve chips operating within a networked system. AMD recognizes this and has equipped the MI250X with innate capabilities for seamless integration into clustered setups.
Through clustering, multiple MI250X chips combine to function as a singular, more powerful unit. Consequently, the MI250X excels in enhancing overall processing capacity. This strategic pairing with other units accentuates its scalability, ensuring it remains adept at handling the escalating demands of the future, particularly in the realm of AI.
6. Software and Compatibility
H100
NVIDIA has long been a pioneer in AI and deep learning. Consequently, the H100 exhibits broad compatibility with a diverse array of AI frameworks. Whether working with TensorFlow, PyTorch, or Caffe, the H100 seamlessly integrates, providing developers with a streamlined experience.
NVIDIA's software toolset, particularly CUDA, proves to be a transformative element. CUDA, serving as a parallel computing platform and programming model, unleashes the full potential of the H100, allowing developers to maximize performance. The wealth of libraries, developer tools, and community support positions CUDA as a preferred choice for many AI professionals.
MI250X
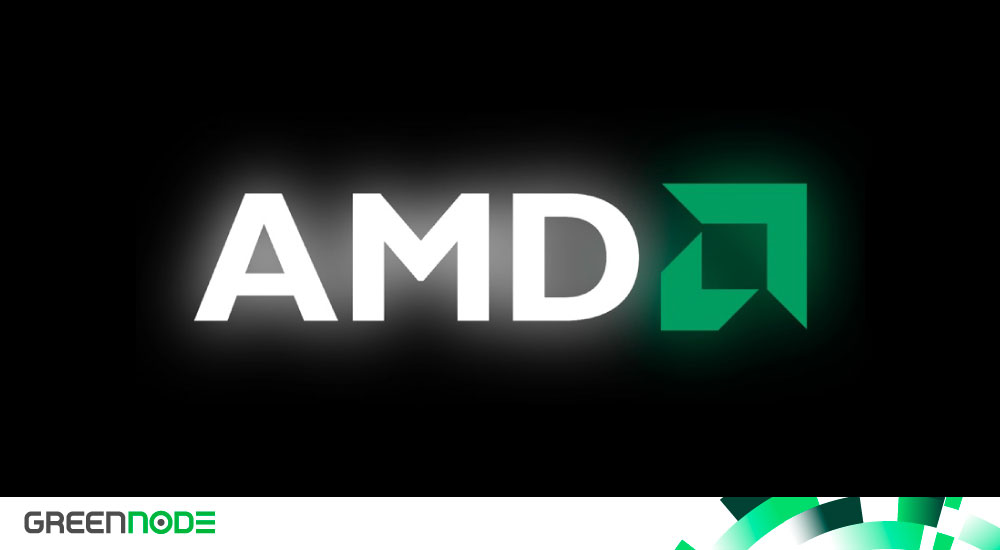
While the MI250X may not exhibit as broad compatibility as the H100, it is intricately integrated into AMD's ecosystem. This integration ensures optimized peak performance when utilized within AMD's environment. AMD's open-source software platform, ROCm (Radeon Open Compute), serves as the counterpart to NVIDIA's CUDA.
Moreover, ROCm is designed to deliver GPU-accelerated computation and features a suite of advanced tools and libraries specifically tailored for the MI250X. Particularly beneficial for specialized tasks within the AMD environment, these tools can provide a competitive advantage over generic solutions. Consequently, individuals working with AI datasets or deploying advanced algorithms within the AMD product suite are likely to favor this chip.
H100 vs. MI250X: 5 Key Insights
- The AMD MI250X outperforms significantly in raw performance benchmarks.
- The NVIDIA H100 boasts enhanced performance with reduced power consumption at only 350W, showcasing superior power efficiency.
- While the H100 is competitive in memory, the MI250X excels in both data retrieval speeds and storage capacity.
- The MI250X provides exclusive access to AMD’s suite of proprietary tools.
- In terms of price, the MI250X offers substantial value, making it a preferred choice for budget-conscious professionals.
Final Thoughts
Choosing between NVIDIA and AMD for AI computing is a challenging decision, considering both companies are global leaders in the field. Both GPUs are cutting-edge, designed to excel in their domains. The decision ultimately depends on your specific needs, operating ecosystem, and budget constraints.
In AI projects, performance metrics are crucial, especially in comparing the H100 and MI250X. The MI250X offers superior and consistent FP32 performance at 47.87 teraFLOPS, while the H100 surpasses in FP32 with 51.22 teraFLOPS but lags slightly in FP64 at 25.61 teraFLOPS. Although MI250X excels in performance, it comes at the cost of efficiency.
Budget becomes the decisive factor. The H100, priced around $30,000, is a significant investment and can be challenging to find. In contrast, the MI250X, priced at roughly $10,000, provides a compelling deal at a third of the cost.
For those with an unrestricted budget and a need for vast compatibility, the H100 is a contender. However, if budget considerations are significant, it's challenging to overlook the MI250X. Ultimately, the choice between H100 and MI250X hinges on individual priorities and specific use cases.
If your business is in search of the H100, GreenNode stands out as a reliable partner of NVIDIA, presenting advanced solutions featuring the formidable NVIDIA H100. As a collaborative force, GreenNode maximizes its partnership with NVIDIA to deliver inventive computing solutions that tap into the exceptional capabilities of the H100, addressing the diverse needs of our clientele.